

He, C., Niu, P., Yang, R., et al.: Incipient rolling element bearing weak fault feature extraction based on adaptive second-order stochastic resonance incorporated by mode decomposition. Hu, M., Wang, G., Ma, K., et al.: Bearing performance degradation assessment based on optimized EWT and CNN.
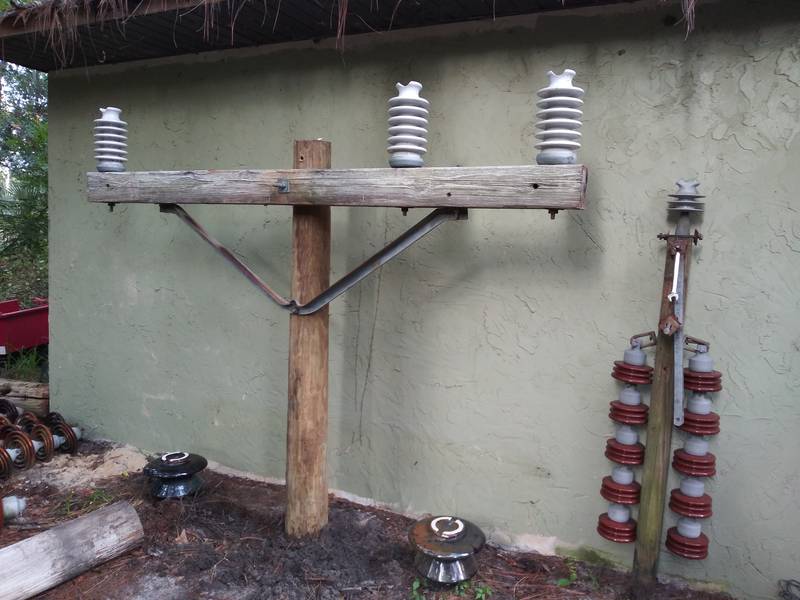
Zhang, Z., Hong, W.C.: Electric load forecasting by complete ensemble empirical mode decomposition adaptive noise and support vector regression with quantum-based dragonfly algorithm. Zhong, T., Qu, J., Fang, X., et al.: The intermittent fault diagnosis of analog circuits based on EEMD-DBN. Li, H., Bu, S., Wen, J.R., et al.: Synthetical modal parameters identification method of damped oscillation signals in power system. Li, M.W., Xu, D.Y., Geng, J., et al.: A ship motion forecasting approach based on empirical mode decomposition method hybrid deep learning network and quantum butterfly optimization algorithm. Wang, X., Si, S., Li, Y.: Hierarchical diversity entropy for the early fault diagnosis of rolling bearing. Jones, D., Snider, C., Nassehi, A., et al.: Characterising the digital twin: a systematic literature review. Peng, B., Xia, H., Lv, X., et al.: An intelligent fault diagnosis method for rotating machinery based on data fusion and deep residual neural network. He, D., Liu, C., Jin, Z., et al.: Fault diagnosis of flywheel bearing based on parameter optimization variational mode decomposition energy entropy and deep learning. Zhang, Z., Shao, M., Ma, C., et al.: An enhanced domain-adversarial neural networks for intelligent cross-domain fault diagnosis of rotating machinery. The result shows that the proposed method has great superiority in the early fault diagnosis of rotating machinery. Two experiments on bearings and gears show that the fault diagnosis accuracy by DMKELM is 99 and 98.5%, respectively, which is at least 1% higher than comparative methods and increases by 4% after noise reduction. Finally, the radial basis kernel function (RBF) and polynomial kernel (PK) are introduced to construct the mixed kernel function, which can enhance the classification performance and generalization ability of the model. Secondly, the optimized VMD (OVMD) with sample entropy is created to reduce noise and reconstruct the signals. Firstly, the improved whale optimization algorithm (IWOA) is designed by introducing the iterative chaotic mapping, nonlinear convergence factor and inertia weight to optimize the VMD parameters. An intelligent early fault diagnosis method for rotating machinery is proposed based on the parameter optimization of the variational mode decomposition (VMD) and deep multi-kernel extreme learning machine (DMKELM). Therefore, it is still a great challenge to make early fault diagnosis. The effect of early fault vibration signals from rotating machinery is weak and easily drowned out by intense noise.
